Unlocking the Power of Data Annotation Tools and Platforms
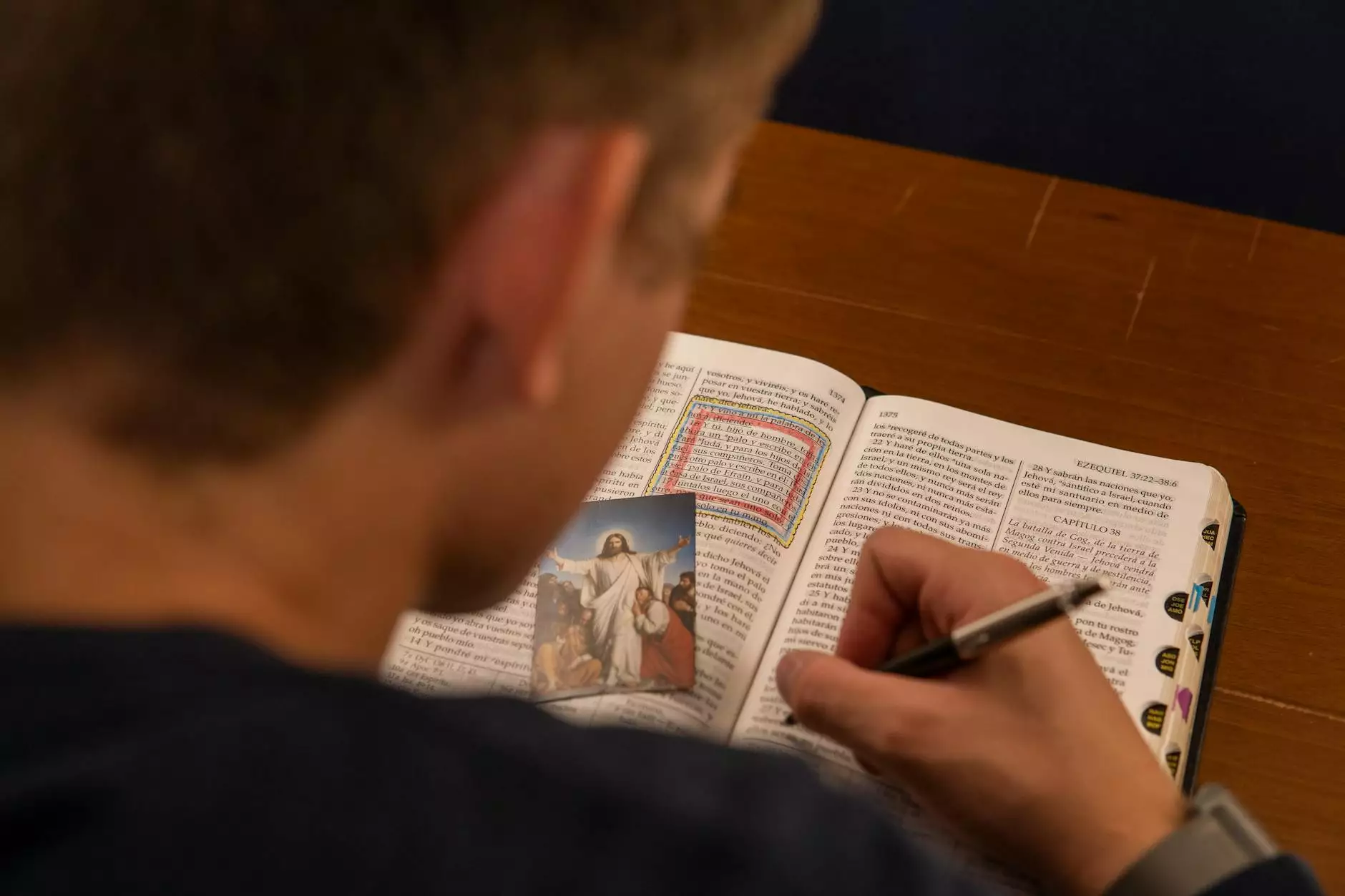
In the digital age, the importance of high-quality datasets has skyrocketed. As organizations increasingly rely on artificial intelligence (AI), machine learning (ML), and data-driven decision-making, the need for precise, well-structured data has never been clearer. This is where data annotation tools and platforms come into play.
What is Data Annotation?
Data annotation is the process of labeling data to make it understandable for AI and ML algorithms. This meticulous work involves categorizing data into recognizable formats so that AI systems can interpret and learn from it effectively. Whether you are dealing with images, video, audio, or text, proper data annotation is critical for training high-performing AI models.
The Importance of Data Annotation Tools
With the growing demand for annotated data, businesses are increasingly turning to data annotation tools for efficient handling of large datasets. Here are several reasons why these tools are essential:
- Efficiency: Manual annotation is time-consuming and can lead to inconsistencies. Automation through data annotation tools speeds up the process and ensures uniformity in labeling.
- Scalability: As data volumes grow, the scalability of your annotation solution becomes vital. Data annotation platforms can handle large datasets without sacrificing quality.
- Accuracy: Advanced algorithms within annotation tools can reduce human error, improving the overall accuracy of labeled data.
- Collaboration: Many data annotation platforms include features that facilitate team collaboration, making it easier to manage annotation projects.
Types of Data Annotation Tools
Data annotation tools come in various forms, each tailored to different types of data:
1. Image Annotation Tools
Image annotation involves labeling objects, segments, or points of interest within images. Common techniques include:
- Bounding Boxes: Drawing rectangles around objects to define their locations.
- Polygonal Annotation: Outlining objects using polygons for more detailed segmentation.
- Semantic Segmentation: Classifying each pixel of the image into predefined categories.
2. Text Annotation Tools
Text annotation tools are used for labeling parts of text data, such as:
- Named Entity Recognition: Identifying and categorizing entities in text, like names, organizations, and locations.
- Sentiment Analysis: Determining the sentiment behind the text (positive, negative, neutral).
- Intent Classification: Understanding the underlying intention behind user queries, especially in chatbots.
3. Audio Annotation Tools
Audio annotation involves the labeling of audio clips for various applications, including speech recognition. Essential techniques include:
- Speech Transcription: Converting spoken language into text accurately.
- Speaker Identification: Labeling audio segments to denote different speakers.
- Emotion Recognition: Annotating audio tones to determine emotional context.
Data Annotation Platforms: A Comprehensive Overview
Data annotation platforms are comprehensive solutions that provide tools, methodologies, and community support for data annotation projects. Investing in a robust platform can streamline your data handling process considerably. Here’s an overview of what to consider when choosing the right data annotation platform:
1. User Interface and Ease of Use
The platform’s user interface should be intuitive and user-friendly. A complex interface can hinder productivity and lead to errors in the annotation process.
2. Supported Data Types
Choose a platform that supports the data formats you work with. Compatibility with multiple data types, such as images, text, video, and audio, will help consolidate your annotation efforts.
3. Integration Capabilities
The platform should integrate seamlessly with your existing workflows. Whether it’s hooking into your data pipeline or sharing annotated data with other applications, integration is key to a smooth operation.
4. Quality Assurance Features
High-quality annotations are crucial for machine learning success. Look for platforms that offer features like quality checks, review processes, and expert validation.
5. Cost-Effectiveness
While investing in a robust annotation platform is essential, managing costs is critical for any business. Evaluate pricing structures to ensure you receive the best value for your investment.
The Future of Data Annotation
The future of data annotation is bright and continuously evolving. With advancements in AI and ML, we can expect:
- Automation: Increasing use of AI-based tools that can perform initial annotations, allowing human annotators to focus on complex cases.
- More Interactive Platforms: Platforms are likely to become increasingly interactive, enabling real-time collaboration and editing.
- Quality Over Quantity: As the understanding of model training improves, emphasis will shift towards the quality of data rather than sheer volume.
Choosing Keylabs AI for Your Data Annotation Needs
If you are looking for a capable partner in data annotation, consider Keylabs AI.
With a sophisticated array of tools designed for every kind of data, they offer:
- Customizable Annotation Solutions: Adapt annotation processes to fit your specific business needs.
- Expertise: Benefit from a team of skilled annotators ready to assist with complex data sets.
- Real-time Collaboration: Boost team productivity with collaborative features that streamline the annotation review process.
Conclusion
Data annotation tools and platforms are vital for maximizing the effectiveness of your AI and ML applications. By investing in high-quality annotation solutions, organizations can significantly improve the accuracy and reliability of their AI models, leading to better decision-making and performance.
Whether you’re looking to enhance image recognition technologies, refine text analysis, or improve audio processing, the right data annotation platform can make all the difference. Explore the capabilities at Keylabs AI and transform your data annotation strategy today!
https://keylabs.ai/