The Ultimate Guide to **Image Data Annotation** for Businesses
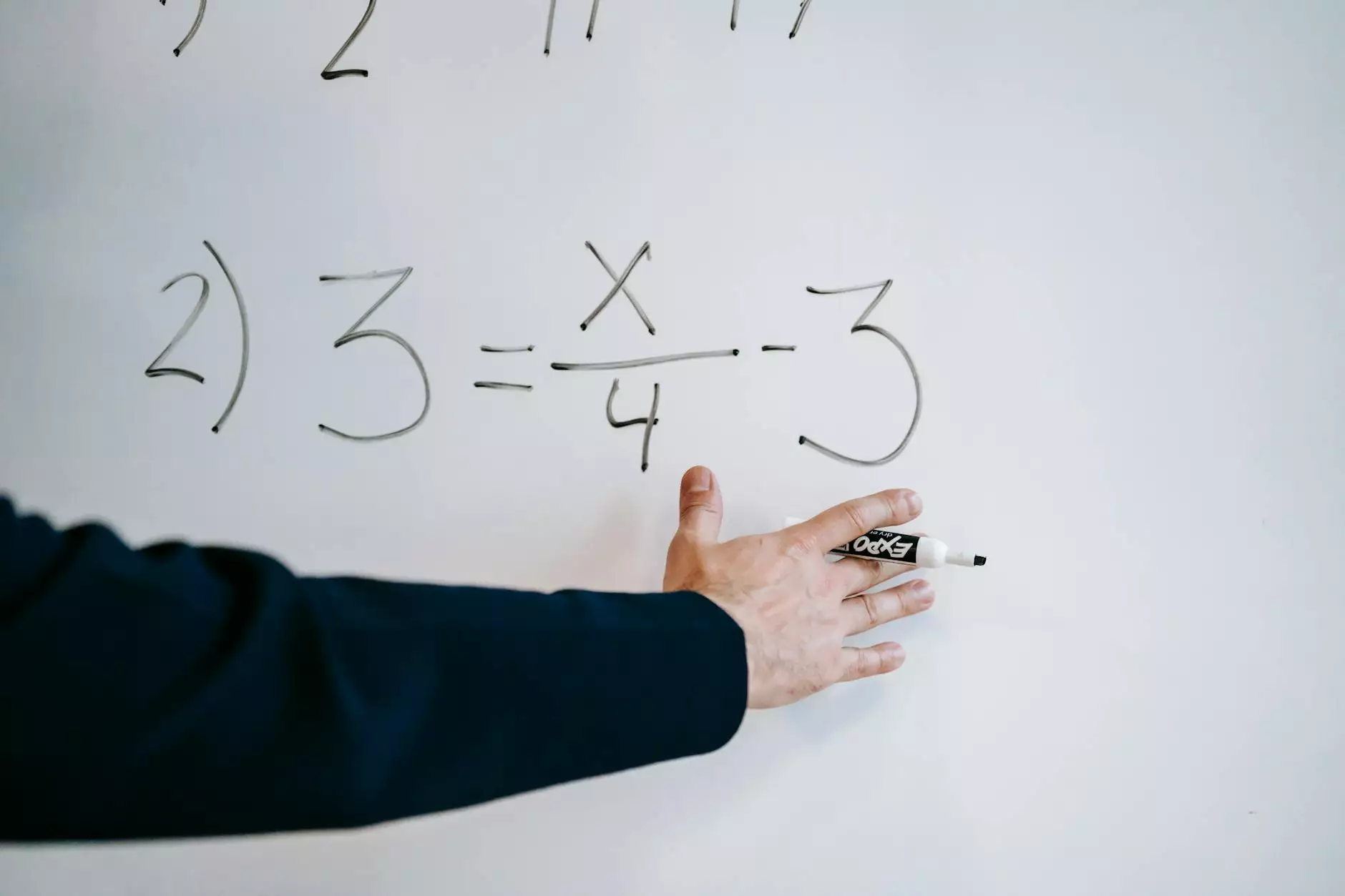
In today's data-driven world, image data annotation has become a crucial element for businesses looking to leverage artificial intelligence (AI) and machine learning (ML) technologies. This detailed article explores the landscape of image data annotation, its significance, and how a robust data annotation tool and data annotation platform can yield measurable benefits for your organization.
Understanding Image Data Annotation
Image data annotation refers to the process of labeling images in order to teach machines to recognize specific attributes and objects. It acts as a foundation for training AI models, particularly in the realm of computer vision. Businesses harness this technology for diverse applications ranging from autonomous vehicles to medical imaging and e-commerce product recommendations.
Importance of Image Data Annotation in Modern Business
As companies increasingly turn to AI to streamline operations, the significance of accurate and high-quality image data annotation cannot be overstated. Here are several reasons why this process is pivotal:
- Enhancing Machine Learning Models: Accurate image annotation is vital for developing precise and efficient machine learning models. The better the data, the better the model's performance.
- Broadening Application Scope: Image data annotation supports various applications, such as object detection, image segmentation, and facial recognition, enabling businesses to innovate continuously.
- Improving User Experience: Annotated images allow businesses to offer personalized recommendations and improve customer interactions, thereby enhancing overall user experience.
- Boosting Automation: Automation systems reliant on AI require annotated images to perform tasks without human intervention, increasing operational efficiency.
The Process of Image Data Annotation
The process of image data annotation can be quite intricate and typically involves several steps:
- Defining Objectives: Before annotating images, businesses must clearly outline the objectives. What do they wish to achieve with machine learning? This stage defines the criteria for annotation.
- Data Collection: Collect a diverse set of images that represent the real-world scenarios you intend to analyze. Ensure the dataset is large enough for a comprehensive training process.
- Choosing Annotation Techniques: Depending on the project, different annotation techniques may be required, including:
- Bounding Boxes: Used for object detection; rectangles are drawn around objects in images.
- Semantic Segmentation: Pixel-level categorization to identify particular areas of an image.
- Image Classification: Labeling entire images according to their content.
- Data Annotation: This step involves the actual labeling of images. It can be performed manually, semi-automatically, or fully automatically, depending on resources.
- Quality Assurance: After annotation, it's crucial to ensure the results meet quality standards. This may involve multiple rounds of reviews and validation.
- Model Training: Once data is annotated, it can be employed to train machine learning models, improving accuracy in live tasks.
Challenges in Image Data Annotation
While image data annotation is essential, it also presents unique challenges:
- Time-Consuming: The process can be labor-intensive, often requiring substantial time and effort, especially for large datasets.
- Cost Implications: High-quality annotation services can be costly; businesses need to balance budget and quality.
- Consistency and Accuracy: Maintaining high levels of accuracy and consistency across annotations is vital yet challenging.
- Scalability Issues: As businesses grow, their data requirements may scale beyond initial capabilities, necessitating flexible annotation solutions.
How Keylabs.ai Addresses Your Data Annotation Needs
At Keylabs.ai, we understand the demands of modern businesses striving to utilize AI technology effectively. Our data annotation tool and data annotation platform are equipped to provide:
- User-Friendly Interface: Our platform is designed with an intuitive interface that simplifies the annotation process, allowing teams to work efficiently.
- High-Quality Annotated Data: We prioritize accuracy and consistency, ensuring that your annotated images will meet the highest standards required for training AI models.
- Scalable Solutions: Whether you're a startup or a large enterprise, our solutions adapt to your growing annotation needs.
- Cost-Efficiency: We offer competitive pricing to ensure you receive exceptional value without compromising on quality.
- Expert Support: Our team of experts is available to provide guidance and support throughout the annotation process, helping you achieve your objectives.
Applications of Image Data Annotation in Various Industries
Wondering where your business might benefit from image data annotation? Here are some examples of how it's applied across various industries:
1. Healthcare
In healthcare, annotated medical images—such as X-rays, MRIs, and CT scans—are vital for training AI algorithms to assist in diagnosing diseases and detecting anomalies.
2. Automotive
In the automotive sector, particularly for autonomous vehicles, accurately annotated images help in training systems to identify and respond to road signs, pedestrians, and other vehicles.
3. Retail and E-commerce
For retailers, implementing image data annotation enables personalized product recommendations and enhanced search functionalities, ultimately increasing sales and customer satisfaction.
4. Agriculture
Farmers use image annotation to monitor crop health and detect pests or diseases, enabling timely interventions that can save resources and maximize yield.
5. Security
In security applications, image data annotation can aid in facial recognition systems to ensure better surveillance and fraud detection mechanisms.
Future Trends in Image Data Annotation
The field of image data annotation is constantly evolving. Here are some trends that businesses should watch:
- Automation and AI: The adoption of sophisticated algorithms is expected to increase, streamlining the annotation process and reducing time expenditures.
- More Interactive Annotation Tools: As technology advances, we can anticipate more interactive tools that allow real-time collaboration and speedier feedback loops.
- Enhanced Accuracy via AI-Assisted Tools: Machine learning tools that assist human annotators may grow more common, enhancing annotation precision and speed.
- Focus on Ethical Data Practices: As data privacy issues gain traction, businesses must prioritize ethical practices in their data annotation methodologies.
Conclusion
In summary, image data annotation is a cornerstone of AI and machine learning applications across various industries. By integrating a reliable data annotation tool and data annotation platform like those offered by Keylabs.ai, businesses can harness the power of machine learning to drive innovation, enhance user experience, and ultimately achieve significant operational efficiencies.
As you embark on your data annotation journey, remember that the quality and accuracy of your labeled images will massively influence your AI outcomes. Choose wisely, invest in quality data annotation, and watch your business thrive in the era of artificial intelligence and machine learning.