The Transformative Impact of Agriculture Datasets for Machine Learning
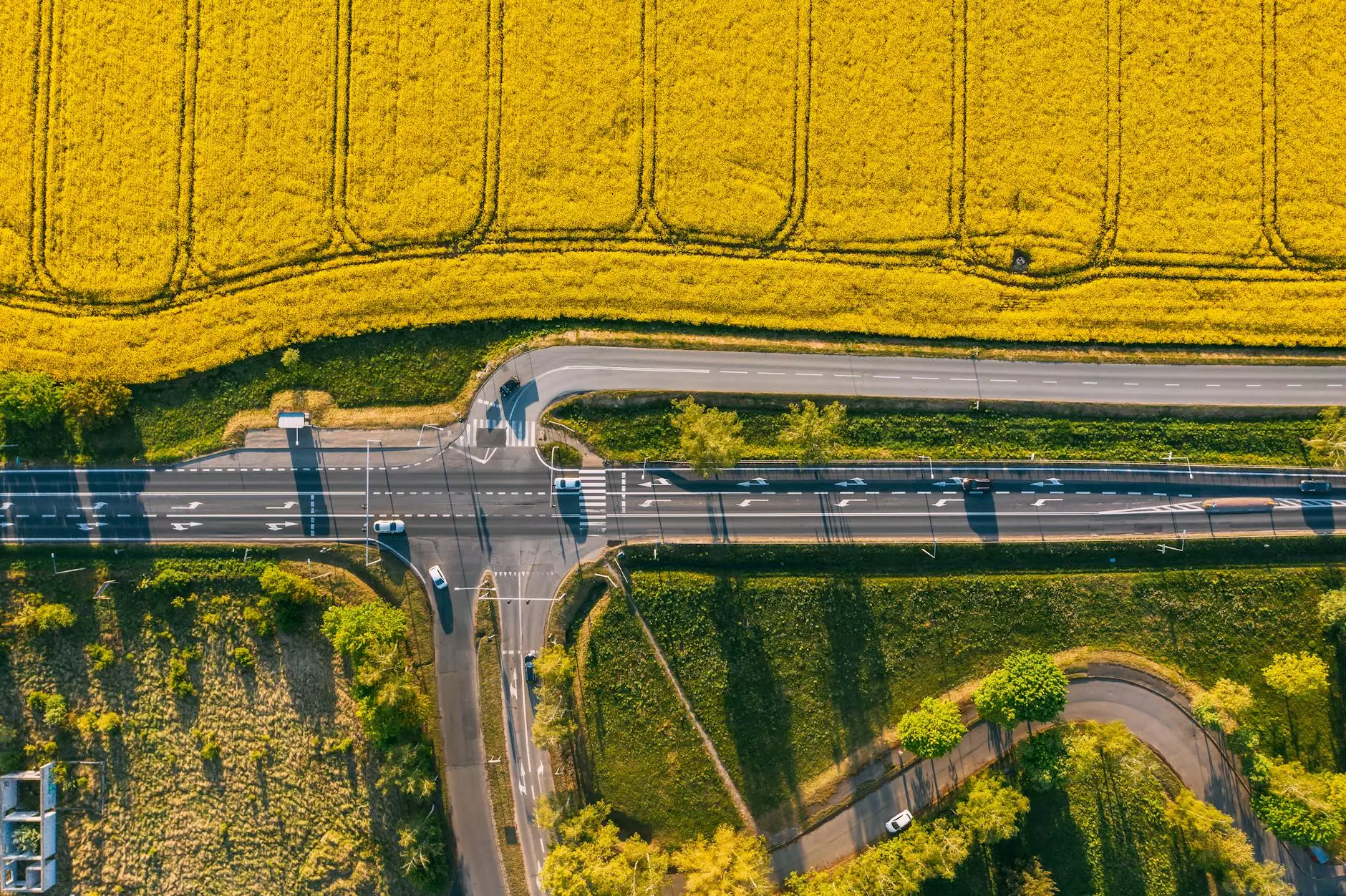
Agricultural innovation has taken a massive leap in recent years, driven by advancements in technology. One of the most significant catalysts for this change is the use of machine learning, which allows for data-driven decision-making in farming. But where does this data come from? The answer lies in agriculture datasets for machine learning, which serve as the backbone of smart agriculture.
Understanding Agriculture Datasets
At its core, an agriculture dataset is a collection of data related to various aspects of farming and agriculture, which can include:
- Crops and yield outcomes
- Soil conditions and fertility
- Weather patterns
- Pest infestations
- Irrigation techniques
- Market prices
- Supply chain logistics
This data is collected from multiple sources, including farms, weather stations, satellite imagery, and research studies. When compiled intelligently, these datasets can provide valuable insights to farmers, researchers, and policy-makers.
The Role of Machine Learning in Agriculture
Machine learning, a subset of artificial intelligence, allows computers to learn from and make predictions based on data. In agriculture, this means analyzing vast amounts of data to uncover patterns and insights that would be impossible to detect with the naked eye. Let's explore some of the key benefits of utilizing agriculture datasets for machine learning.
1. Enhanced Yield Prediction
One of the primary applications of machine learning in agriculture is yield prediction. By analyzing historical yield data along with weather patterns and soil conditions, algorithms can predict future crop yields with remarkable accuracy. This predictive capability helps farmers make more informed decisions about:
- Crop selection
- Planting and harvesting times
- Resource allocation
2. Precision Farming
Precision farming involves using technology and data to manage agricultural practices more effectively. With the help of agriculture datasets, farmers can employ machine learning to:
- Optimize irrigation and fertilization
- Monitor crop health via remote sensors
- Reduce waste by applying inputs only when necessary
This approach not only improves efficiency but also minimizes environmental impact.
3. Pest and Disease Management
Pests and diseases can decimate crops if not managed promptly. Leveraging datasets that include historical pest and disease incidence, machine learning models can predict outbreaks and suggest preventive measures. This proactive approach helps in:
- Timely intervention
- Reduced chemical usage
- Improved crop health
4. Supply Chain Optimization
Understanding the agricultural supply chain is critical for ensuring food security and minimizing costs. With machine learning analyzing agriculture datasets, stakeholders can optimize:
- Logistics and transportation
- Inventory management
- Market demand forecasting
This leads to a more efficient distribution of resources and goods.
Sources of Agriculture Datasets
When it comes to agriculture datasets, the variety of available data sources is vast. Here are some of the primary sources to consider:
1. Government Agencies
Many governments collect and publish agricultural data through departments of agriculture. This can include:
- Census data
- Research publications
- Statistical reports
2. Satellite and Remote Sensing
Satellites capture vast amounts of data on land use, crop health, and environmental conditions. This remote sensing technology provides:
- High-resolution imagery
- Timely updates on asset conditions
- Data for modeling climate impacts
3. IoT Devices
The Internet of Things (IoT) has emerged as a game-changer in agriculture. Sensors placed in fields can gather real-time data on:
- Soil moisture levels
- Temperature and humidity
- Crop growth metrics
4. Academic and Research Institutions
Research institutions often conduct studies and publish datasets that are beneficial for machine learning applications in agriculture. Collaboration between industry and academia can lead to:
- Innovative solutions
- Access to proprietary data
- Advancements in agricultural practices
Challenges in Utilizing Agriculture Datasets
While the benefits of using agriculture datasets for machine learning are numerous, several challenges exist that must be addressed:
1. Data Quality
The accuracy of machine learning predictions largely depends on the quality of the underlying data. Inconsistent or flawed data can lead to poor model outcomes. Ensuring data quality involves:
- Regular audits of data sources
- Standardization of data formats
- Employing validation techniques
2. Data Privacy and Security
As farming becomes increasingly data-driven, concerns about privacy and security arise. It’s essential to establish protocols for:
- Data sharing
- Access control
- Data ownership rights
3. Integration of Diverse Data Sources
Combining data from various sources can be challenging due to differences in formats and standards. Developing effective integration strategies is crucial for maximizing the insights gained from agriculture datasets.
Conclusion: The Future of Agriculture with Machine Learning
The application of agriculture datasets for machine learning presents an exciting opportunity to transform the agricultural sector. By harnessing the power of technology, farmers can optimize practices, enhance yields, and contribute to sustainable farming. As we move forward, addressing the challenges and continuing to innovate will be key to unlocking the full potential of data-driven agriculture.
As we embrace the future, companies like keymakr.com can play a critical role in supporting the agricultural community through services that enhance data management, analysis, and security for farmers and stakeholders alike. By focusing on these critical areas, we can pave the way for a more efficient, sustainable, and prosperous agricultural landscape.
agriculture dataset for machine learning